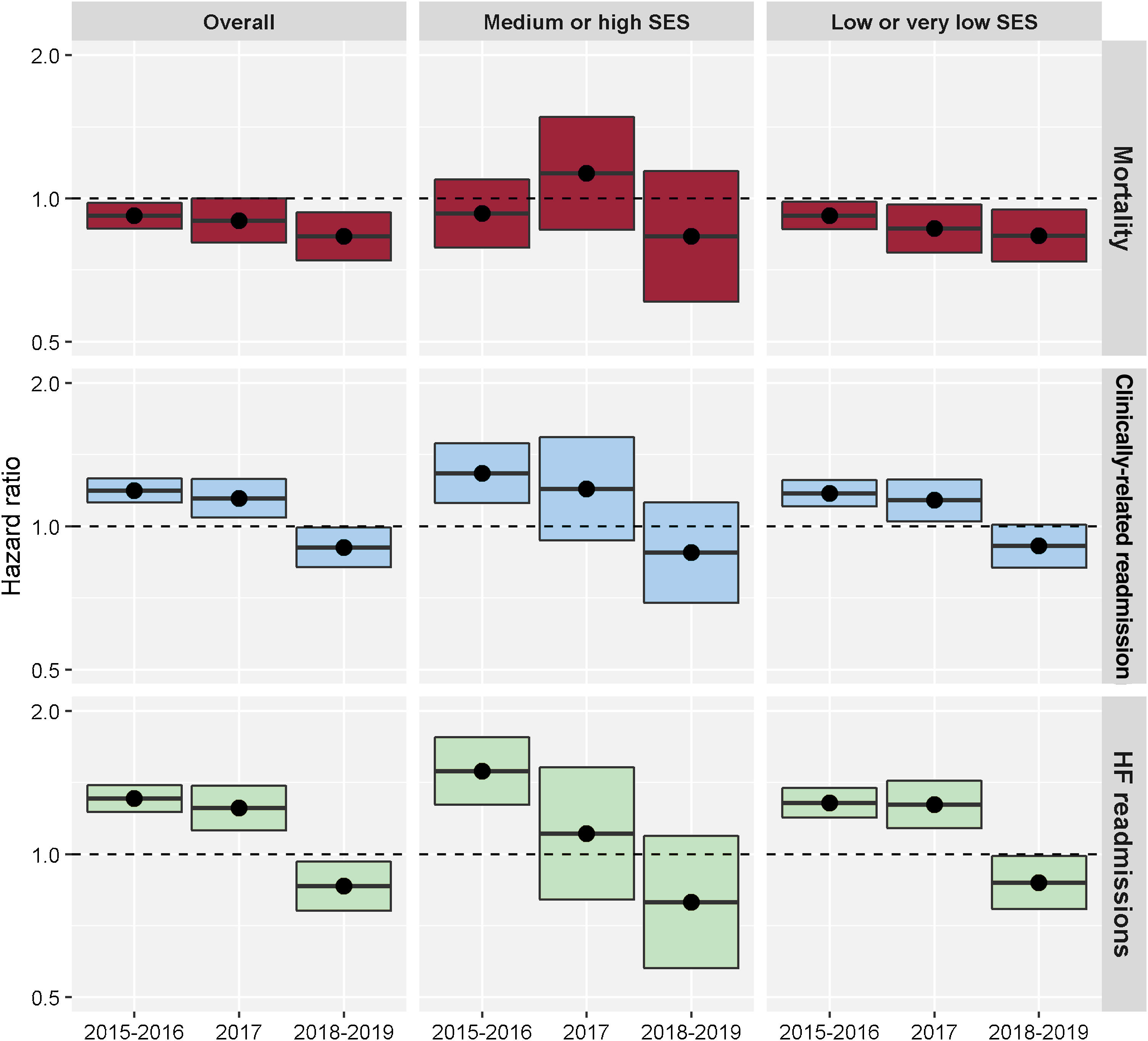
Low socioeconomic status (SES) is associated with poor outcomes in patients with heart failure (HF). We aimed to examine the influence of SES on health outcomes after a quality of care improvement intervention for the management of HF integrating hospital and primary care resources in a health care area of 209 255 inhabitants.
MethodsWe conducted a population-based pragmatic evaluation of the implementation of an integrated HF program by conducting a natural experiment using health care data. We included all individuals consecutively admitted to hospital with at least one ICD-9-CM code for HF as the primary diagnosis and discharged alive in Catalonia between January 1, 2015 and December 31, 2019. We compared outcomes between patients exposed to the new HF program and those in the remaining health care areas, globally and stratified by SES.
ResultsA total of 77 554 patients were included in the study. Death occurred in 37 469 (48.3%), clinically-related hospitalization in 41 709 (53.8%) and HF readmission in 29 755 (38.4%). On multivariate analysis, low or very low SES was associated with an increased risk of all-cause death and clinically-related hospitalization (all Ps <.05). The multivariate models showed a significant reduction in the risk of all-cause death (HR, 0.812; 95%CI, 0.723-0.912), clinically-related hospitalization (HR, 0.886; 95%CI, 0.805-0.976) and HF hospitalization (HR, 0.838; 95%CI, 0.745-0.944) in patients exposed to the new HF program compared with patients exposed to the remaining health care areas and this effect was independent of SES.
ConclusionsAn intensive transitional HF management program improved clinical outcomes, both overall and across SES strata.
Keywords
Heart failure (HF) is a life-threatening condition with a significant influence on mortality, morbidity, and health-related quality of life in affected individuals. The increased prevalence of HF, as well as rising medical resource consumption and associated costs, represent a challenge to health care systems.1–5
The management of HF is complex and often requires many resources since it involves the combination of multiple therapeutic, structural, and educational interventions in multiple levels of care and provided by multidisciplinary teams.6–14 In this regard, HF programs receive an IA level of recommendation in international clinical guidelines.14
In the context of managed care, low socioeconomic status (SES) and other psychosocial determinants,15 have been identified as factors linked to decreased life expectancy and an increased burden of unplanned hospitalizations in patients with HF3.
In this regard, some studies have attempted to ascertain how low SES may moderate the efficacy and effectiveness of self-management strategies in multidisciplinary team interventions for chronic conditions.16 However, only a few of these studies have focused on HF care-orientated programs and none have evaluated the influence of SES on hard endpoints.16
Thus, little is known about the influence of SES on the health outcomes of patients exposed to specialized multidisciplinary integrated HF programs. In particular, it is not known whether the disparity in outcomes determined by SES inequalities can be mitigated by intensive HF management in integrated care programs.
Understanding the influence of SES in the clinical outcomes of patients with HF living in a universal health care system could help identify vulnerable patient subgroups who may require specific interventions. This knowledge gap needs to be addressed, particularly in the context of the implementation of multidisciplinary intervention programs aiming to improve the care of patients with HF in integrated care settings.16
Given the above-mentioned knowledge gap, the present study was designed to address the impact of low SES on the effectiveness of multidisciplinary, nurse-led, transitional care heart failure programs integrating primary care and hospital resources for patients with chronic HF with a recent HF hospitalization.
To address this objective, we first implemented a transitional, multidisciplinary, nurse-based HF program in a particular integrated health care area; second, we conducted a pragmatic population-based evaluation of its implementations; and third, we evaluated the influence of SES on the effectiveness of the care delivered to patients in the setting of the new HF program.
METHODSAdditional information on study context, data collection, research methods, study design, inclusion and exclusion criteria, study endpoints, and statistical aspects of the study are provided in sections A-F of the online supplementary data.
The study was conducted in Catalonia (N=7 816 419 as of 2019) where universal public health care coverage is granted by law. In recent years, the Catalan Health Service (CatSalut) has promoted several quality of care improvement initiatives for patients with chronic HF.6,7,17–20 For more study context information see section A of the supplementary data.
Since 2017, a new program to improve quality of care of patients with chronic HF has been designed and implemented in Bellvitge University Hospital (HUB) and the Delta del Llobregat Primary Care Service (DELTA) integrated health care area (209 255 inhabitants). The HUB-DELTA HF program was designed as a nurse-based multidisciplinary, transitional care HF program based on the conceptual framework provided by the Chronic Care Model including all components of care and interventions that have shown benefits in the care of patients with HF.6,10,11,13,14,21,22 This model was successfully implemented previously in a different health care area.7 In the current implementation, the model has been updated and improved in several aspects described in section B of the supplementary data.
Data sources and data quality controlSince 2011, the Health Department of the Government of Catalonia has used an automated administrative health care database (the Catalan Health Surveillance System [CHSS]), which periodically collects detailed individual-level information on demographics and socioeconomic characteristics, as well as exhaustive health-related and medical resource use information generated by the interactions between Catalan residents and the public health care system. This longitudinal, quality-controlled, updated information system allows the performance of epidemiological analyses, evaluations of health care interventions, and public analysis and benchmarking of health indicators across health care areas, among other assessments.1–3,23–25
This database integrates information from several sources, including information on citizens’ vital status (National Statistics Institute, Spanish Statistical Office). Further details on the data sources used in this study are described in section C of the supplementary data.
Study design, study population, coding criteria, and ethicsThe primary objective of the study was to determine whether the effectiveness of an integrated HF program may differ according to patient’ SES. In other words, whether the disparity in outcomes observed across SES levels can be mitigated with more intensive integrated care models. Secondary objectives were: a) to confirm the association between SES and outcomes in the HF population of Catalonia, and b) to evaluate the effectiveness of the implementation of a transitional HF program integrating hospital and primary care resources in a pragmatic evaluation (natural experiment) using population-based health care data.
To evaluate the influence of SES on the effectiveness of a multilevel, multidisciplinary, transitional care program for the management of patients with HF we followed 3 critical steps.
In the first step, we designed and implemented a comprehensive HF program in the HUB-DELTA integrated health care area between November 2016 and December 2019. We included 3 distinct implementation periods: preimplementation period (2015 and 2016), transition period (2017) and consolidation of the implementation period (2018 and 2019).
As a second step, we designed a pragmatic, population-based evaluation of the implementation of the program by conducting a natural experiment. For the purposes of this study, we included all individuals consecutively admitted to hospital with at least one ICD-9-CM code for HF as the primary diagnosis and discharged alive in Catalonia between January 1, 2015 and December 31, 2019. The ICD-9-CM codes used for hospital admission due to HF were the following: 398.91, 402.x1, 404.x1, 404.x3, 428.0, 428.1, 428.2x, 428.x3, and 428.x4. In all patients, SES, general clinical characteristics, demographic information, information on comorbidities and previous medical resource use were obtained at baseline. Further details on complete coding criteria are provided in section D of the supplementary data.
Clinical outcomes were measured and analyzed for all patients between January 1, 2015 and December 31, 2019. For the index admission at each time period or year of analysis and successive clinically-related and HF readmissions, we considered only unplanned acute admissions lasting more than 24hours.
The effectiveness of the implementation of the program was measured at 2 levels: first, by comparing the outcomes of patients exposed to the HUB-DELTA HF program between implementation periods, taking 2015 (preimplementation) as the reference year and the period from 2015 to 2016 (preimplementation period) as the reference period (intragroup comparison) and, second, by comparing outcomes between patients in the HUB-DELTA area with those in the remaining areas of CatSalut at each predefined implementation period (between-group comparisons).
The third step of the current project involved the evaluation of the effectiveness of the HUB-DELTA HF program stratified according to the level of SES across the studied time periods.
The primary outcome variable of the study was the time until the first clinically-related readmission. Secondary outcome variables were time until the first admission for HF and time to death.
The present study was conducted in accordance with the Declaration of Helsinki. All data were handled according to the General Data Protection Regulation 2016/679 on data protection and privacy for all individuals within the European Union and the local regulatory framework regarding data protection. The study was approved by the independent ethics committee of the HUB and the Bellvitge Biomedical Research Institute, which waived the need to obtain informed consent for the use of health care data.
Assessment of individual socioeconomic statusThe method used to assess individual SES has been previously reported by our group.3,23 For the purpose of this study, we defined 4 individual income categories according the CHSS database information available on individual annual income: an annual income> €100 000 was considered “high” income, €18 000 to 100 000 was considered “medium” income, and <€18 000 was considered “low” income. These 3 categories included both active workers as well as retired individuals receiving a retirement pension. Finally, individuals who received welfare support by the Government were considered to have “very low income”. For the present analysis, we grouped patients with medium and high income into a single category.
Assessment of other covariates, quality of care indicators, and study endpointsInformation on relevant covariates including age, sex, and comorbidities available at the time of the index admission was used for all patients. Specifically for comorbidities, we used the “adjusted morbidity groups” (GMA [Catalan acronym for “Grups de Morbiditat Ajustats”]) comorbidity classification system.3,26,27 To measure the quality, complexity, and intensity of the new HF program, we used the Heart Failure Intervention Score,28 and the Heart Failure Disease Management Scoring Instrument.29 Clinically-related hospital readmissions were defined using the Chronic Condition Indicator criteria for the ICD-9-CM of the Agency for Healthcare Research and Quality.30 Further details are provided in section E of the supplementary data.
Statistical analysesA detailed description of the statistical methods is provided in section F of the supplementary data. Descriptive statistics were conducted using standard methods.
Several multivariate adjusted Cox proportional hazards models were constructed to analyze, a) the influence of SES on the clinical endpoints of the study in the global population, b) the effect on outcomes of the implementation of the new HF program in the HUB-DELTA health care area, and c) the impact on clinical outcomes across years and predefined time periods according to health care setting (HUB-DELTA area vs the rest of CatSalut) and stratified by SES. In addition, the effect of the interaction between individual SES and health care area (HUB-DELTA vs the rest of CatSalut) across study periods was further explored in similar models.
All models were adjusted for age, sex, individual SES, previous hospitalization, comorbidities (using the GMA morbidity index) and time since diagnosis of HF.
All statistical tests and confidence intervals (CI) were constructed with a type I error alpha level of 5%, with no adjustments for multiplicity. P values below .05 were considered statistically significant. All analyses were performed using R software (version 4.0.2; R Foundation for Statistical Computing, Vienna, Austria).
RESULTSThe study cohort consisted of 77 554 patients discharged alive with a diagnosis of HF between January 1, 2015 and December 31, 2019: 3 396 were exposed to the HUB-DELTA health care area and 74 158 were exposed to the rest of the health care areas in Catalonia. A flowchart of patient inclusion in the study is provided in figure 1 of the supplementary data.
Baseline characteristics of patientsBaseline characteristics of the study sample, both overall and according to health care area (HUB-DELTA vs the rest of Catalonia) are presented in table 1. According to individual annual income, the patients were classified as very low SES (3 535, 4.56%), low SES (61 967, 79.9%), medium SES or high SES (12 018, 15.5%). There were no significant differences in SES between patients in the HUB-DELTA area and the rest of Catalonia. Patients in the HUB-DELTA area tended to be younger and had a lower morbidity burden, as defined by the GMA index and risk levels. The prevalence of cardiovascular (CV) comorbidities did not differ between the 2 groups. However, the burden of previous hospitalizations was higher among the HUB-DELTA patients. As shown in tables 1 and 2 of the supplementary data, the SES distribution of patients tended to improve between January 1, 2015 and December 31, 2019.
Baseline characteristics including the socioeconomic status of patients discharged alive with a primary diagnosis of HF overall and according to health care areas (rest of CatSalut vs HUB-DELTA) between January 1, 2015, and December 31, 2019
Overall | Rest of CatSalut | HUB-DELTA Area | P | |
---|---|---|---|---|
N=77 554 | n=74 158 | n=3396 | ||
Individual annual income | .074 | |||
Medium or high SES | 12 018 (15.5) | 11 468 (15.5) | 550 (16.2) | |
Low SES | 61 967 (79.9) | 59 298 (80.0) | 2669 (78.6) | |
Very low SES | 3535 (4.56) | 3358 (4.53) | 177 (5.21) | |
Sex | .347 | |||
Male | 35 895 (46.3) | 34 296 (46.2) | 1599 (47.1) | |
Female | 41 659 (53.7) | 39 862 (53.8) | 1797 (52.9) | |
Age | 79.8±10.8 | 79.8±10.8 | 79.4±9.96 | .018 |
Age group, y | <.001 | |||
15-49 | 1212 (1.56) | 1172 (1.58) | 40 (1.18) | |
50-64 | 6009 (7.75) | 5766 (7.78) | 243 (7.16) | |
65-74 | 12 372 (16.0) | 11 788 (15.9) | 584 (17.2) | |
75-84 | 28 054 (36.2) | 26 687 (36.0) | 1367 (40.3) | |
>84 years | 29 876 (38.5) | 28 714 (38.7) | 1162 (34.2) | |
Morbidity burden (GMA) | 37.9±16.4 | 37.9±16.4 | 37.0±15.8 | .001 |
Risk levels (GMA) | .004 | |||
Low risk | 1793 (2.31) | 1727 (2.33) | 66 (1.94) | |
Intermediate risk | 12 188 (15.7) | 11 628 (15.7) | 560 (16.5) | |
High risk | 32 138 (41.4) | 30 656 (41.3) | 1482 (43.6) | |
Very high risk | 31 435 (40.5) | 30 147 (40.7) | 1288 (37.9) | |
Years since HF diagnosis | .252 | |||
<1 | 32 738 (42.2) | 31 354 (42.3) | 1384 (40.8) | |
1-2 | 13 396 (17.3) | 12 809 (17.3) | 587 (17.3) | |
3-5 | 14 986 (19.3) | 14 294 (19.3) | 692 (20.4) | |
> 5 | 16 434 (21.2) | 15 701 (21.2) | 733 (21.6) | |
Previous MI | 15 794 (20.4) | 15 129 (20.4) | 665 (19.6) | .255 |
Atrial fibrillation | 28 780 (37.1) | 27 559 (37.2) | 1221 (36.0) | .159 |
Peripheral vascular disease | 19 196 (24.8) | 18 266 (24.6) | 930 (27.4) | <.001 |
Hypertension | 70 649 (91.2) | 67 554 (91.2) | 3095 (91.2) | 1.000 |
Obesity | 25 945 (33.5) | 24 849 (33.5) | 1096 (32.3) | .141 |
Smoking | 17 326 (22.3) | 16 517 (22.3) | 809 (23.8) | .036 |
Hyperlipidemia | 31 593 (40.7) | 30 162 (40.7) | 1431 (42.1) | .093 |
Diabetes mellitus | 39 684 (51.2) | 37 951 (51.2) | 1733 (51.0) | .861 |
CKD | 41 498 (53.5) | 39 597 (53.4) | 1901 (56.0) | .004 |
Anemia | 43 515 (56.1) | 41 675 (56.2) | 1840 (54.2) | .022 |
COPD | 32 703 (42.2) | 31 263 (42.2) | 1440 (42.4) | .808 |
Cancer | 11 994 (15.5) | 11 419 (15.4) | 575 (16.9) | .017 |
Osteoarthritis | 10 218 (13.2) | 9857 (13.3) | 361 (10.6) | <.001 |
Severe cognitive impairment | 5904 (7.62) | 5710 (7.71) | 194 (5.71) | <.001 |
Cirrhosis | 1657 (2.14) | 1580 (2.13) | 77 (2.27) | .635 |
Major mental health disorder | 9501 (12.3) | 9090 (12.3) | 411 (12.1) | .808 |
Alcohol abuse | 9201 (11.9) | 8556 (11.5) | 645 (19.0) | <.001 |
Opioid abuse | 510 (0.66) | 498 (0.67) | 12 (0.35) | .033 |
Cocaine abuse | 348 (0.45) | 335 (0.45) | 13 (0.38) | .648 |
Number of previous hospital admissions | 1.13±1.59 | 1.12±1.60 | 1.22±1.55 | <.001 |
Number of days in hospital (previous) | 9.65±16.0 | 9.66±16.0 | 9.42±15.6 | .377 |
Number of days in psychiatric unit (previous) | 0.03±1.48 | 0.03±1.50 | 0.03±0.91 | .769 |
Number of days in skilled nursing facility (previous) | 3.19±18.2 | 3.21±18.2 | 2.78±18.8 | .196 |
Number of days in nursing home (previous) | 27.1±92.0 | 27.7±92.9 | 15.0±69.6 | <.001 |
CKD, chronic kidney disease; COPD, chronic obstructive pulmonary disease; GMA, adjusted morbidity groups; HF, heart failure; MI, myocardial infarction; SES, socioeconomic status.
The data are presented as mean±standard deviation or No. (%).
In table 3 of the supplementary data, we present the baseline characteristics of patients in Catalonia between January 1, 2015 and December 31, 2019, according to SE). As shown in tables 3 and 4 of the supplementary data, female sex, older age, and higher comorbidity burden were more prevalent among low or very low SES categories.
During the study period, 55 886 (72.1%) patients experienced at least 1 major adverse event. Death occurred in 37 469 (48.3%), clinically-related hospitalization in 41 709 (53.8%), and HF readmission in 29 755 (38.4%).
In both unadjusted and adjusted analyses, low or very low SES was associated with an increased risk of all-cause death, clinically-related hospitalization, and HF readmission during the study period (table 2). The association of low or very low SES with all-cause death was stronger in the 2015 to 2016 period (HR, 1.086; 95%CI, 1.042-1,133; P <.001). The association with clinically-related admissions (HR, 1.104; 95%CI, 1.039-1,173; P=.001) and HF admissions (HR, 1.154; 95%CI, 1.070-1.244; P <.001) was stronger in the study period corresponding to 2017.
Proportion of occurrence of events according to socioeconomic status (SES) strata after discharge and multivariate (adjusted) Cox proportional hazards models analyzing the risk of events in patients discharged alive with a primary diagnosis of HF in Catalonia between January 1, 2015 and December 31, 2019
Very low SES | Low SES | Medium/high SES | Low/very low vs medium/high SES | |||
---|---|---|---|---|---|---|
Period | No. (%) | No. (%) | No. (%) | HR | 95%CI | P |
All-cause death | ||||||
2015-2016 | 1496 (16.4) | 24 884 (22.1) | 4174 (20.0) | 1.086 | 1.042-1.133 | <.001 |
2017 | 663 (19.3) | 11 571 (21.1) | 2340 (18.8) | 1.083 | 1.015-1.157 | .017 |
2018-2019 | 1376 (17.6) | 25 512 (21.9) | 5504 (20.2) | 1.049 | 0.991-1.110 | .100 |
Clinically-related readmission | ||||||
2015-2016 | 1496 (45.4) | 24 884 (40.5) | 4174 (38.1) | 1.082 | 1.039-1.128 | <.0001 |
2017 | 663 (44.7) | 11 571 (37.2) | 2340 (34.4) | 1.104 | 1.039-1.173 | .001 |
2018-2019 | 1376 (39.2) | 25 512 (36.4) | 5504 (33.7) | 1.092 | 1.040-1.146 | <.0001 |
HF readmission | ||||||
2015-2016 | 1496 (32.9) | 24 884 (29.6) | 4174 (25.6) | 1.120 | 1.067-1.177 | <.0001 |
2017 | 663 (32.7) | 11 571 (26.0) | 2340 (21.4) | 1.154 | 1.070-1.244 | <.0001 |
2018-2019 | 1376 (27.3) | 25 512 (25.5) | 5504 (22.6) | 1.104 | 1.040-1.171 | .0001 |
Models were adjusted for SES, sex, age, previous hospitalization, morbidity index (GMA: associated morbidity groups) and time since diagnosis of HF. No. corresponds to exposed patients and % to the rate of occurrence of events. HF, heart failure; SES, socioeconomic status.
These findings were confirmed in multivariate analyses considering the whole study period between January 1, 2015 and December 31, 2019 (table 5 of the supplementary data). In these adjusted Cox proportional hazards models, low and very low SES were independently associated with all-cause death (HR, 1.071; 95%CI, 1.039-1.104, and HR, 1.1576; 95%CI, 1.092-1.226, respectively), clinically-related readmission (HR, 1.087; 95%CI, 1.057-1.118 and HR, 1.179; 95%CI, 1.121-1.240, respectively), and HF hospitalization (HR, 1.119; 95%CI, 1.082-1.158 and HR, 1.220; 95%CI, 1.149-1.295, respectively).
Effectiveness of the implementation of the HUB-DELTA HF programThe scores obtained by the new HF program in the Heart Failure Disease Management Scoring Instrument and the Heart Failure Intervention Score were 33 (out of 34) and 197 (out of 198), respectively, indicating that the highest possible level of quality of care indicators of delivered care was achieved after the implementation of the program.
Adjusted Cox proportional hazards analyses showed that clinically-related readmission (HR, 0.870; 95%CI, 0.851-0.891; P <.001), HF readmission (HR, 0.846; 95%CI 0.824-0.870; P <.001), and all-cause death (HR, 0.949; 95%CI, 0.925-0.975; P <.001) were all reduced in the HUB-DELTA area in the consolidation period compared with the preimplementation period (table 3 and figure 1).
Multivariate (adjusted) Cox proportional hazards analyses exploring the effect on outcomes of the implementation of the primary care hospital integrated heart failure program in the HUB-DELTA health care area between January 1, 2015, and December 31, 2019
Outcomes in the HUB-DELTA area compared with the reference year (2015) or reference period | HUB-DELTA area vs the rest of CatSalut foreach year or period | |||||
---|---|---|---|---|---|---|
Period | HR | 95%CI | p | HR | 95%CI | P |
All-cause death | ||||||
2015 | 1 | – | – | 0.954 | 0.879-1.035 | .255 |
2016 | 0.905 | 0.880-0.931 | <.001 | 0.897 | 0.815-0.987 | .025 |
2017 | 0.887 | 0.861-0.914 | <.001 | 0.913 | 0.821-1.016 | .096 |
2018 | 0.918 | 0.889-0.949 | <.001 | 0.818 | 0.715-0.937 | .004 |
2019 | 0.883 | 0.847-0.920 | <.001 | 0.778 | 0.619-0.978 | .032 |
2015-2016 | 1 | – | – | 0.929 | 0.873-0.988 | .020 |
2017 | 0.930 | 0.905-0.955 | <.001 | 0.913 | 0.821-1.016 | .096 |
2018-2019 | 0.949 | 0.925-0.975 | <.001 | 0.812 | 0.723-0.912 | <.001 |
Clinically-related readmissions | ||||||
2015 | 1 | – | – | 1.257 | 1.161-1.360 | <.001 |
2016 | 0.997 | 0.970-1.025 | .830 | 1.220 | 1.120-1.328 | <.001 |
2017 | 0.894 | 0.869-0.920 | <.001 | 1.180 | 1.075-1.295 | <.001 |
2018 | 0.898 | 0.871-0.925 | <.001 | 0.890 | 0.794-0.997 | .045 |
2019 | 0.826 | 0.798-0.855 | <.001 | 0.857 | 0.717-1.023 | .088 |
2015-2016 | 1 | – | – | 1.239 | 1.169-1.313 | <.001 |
2017 | 0.895 | 0.873-0.918 | <.001 | 1.180 | 1.075-1.295 | <.001 |
2018-2019 | 0.870 | 0.851-0.891 | <.001 | 0.886 | 0.805-0.976 | .014 |
HF readmissions | ||||||
2015 | 1 | – | – | 1.315 | 1.202-1.438 | <.001 |
2016 | 0.972 | 0.941-1.004 | .082 | 1.415 | 1.287-1.555 | <.001 |
2017 | 0.858 | 0.830-0.888 | <.001 | 1.282 | 1.152-1.427 | <.001 |
2018 | 0.866 | 0.836-0.897 | <.001 | 0.840 | 0.730-0.968 | .016 |
2019 | 0.788 | 0.756-0.821 | <.001 | 0.812 | 0.652-1.011 | .063 |
2015-2016 | 1 | – | – | 1.361 | 1.275-1.452 | <.001 |
2017 | 0.870 | 0.844-0.897 | <.001 | 1.282 | 1.152-1.427 | <.001 |
2018-2019 | 0.846 | 0.824-0.870 | <.001 | 0.838 | 0.745-0.944 | .003 |
Models were adjusted for SES, sex, age, previous hospitalization, morbidity index (GMA, associated morbidity groups) and time since diagnosis of HF. HF, heart failure; SES, socioeconomic status.
Central Illustration. Graphical representation of HR and 95%CI from multivariable (adjusted) Cox proportional models evaluating the impact on clinical outcomes across predefined time periods according to health care setting (HUB-DELTA area vs the rest of CatSalut) overall and by SES strata. Multivariate models showed a significant reduction in the risk of all-cause death (HR, 0.812; 95%CI, 0.723-0.912), clinically-related hospitalization (HR, 0.886; 95%CI, 0.805-0.976) and HF hospitalization (HR, 0.838, 95%CI; 0.745-0.944) in patients exposed to the new HF program built in the HUB-DELTA integrated health care area compared with patients exposed to the remaining health care areas and this effect was independent of SES. The size effect for prevention of hospitalization was more prominent among patients with a medium or high SES. HF, heart failure; HR, hazard ratio; SES, socioeconomic status.
Furthermore, the risk of clinically-related readmission (HR, 0.886; 95%CI, 0.805-0.976; P=.014), HF readmission (HR, 0.838; 95%CI, 0.7451-0.944; P=.003), and all-cause death (HR, 0.812; 95%CI 0.723-0.912; P <.001) was significantly lower in the HUB-DELTA area than in the rest of CatSalut in adjusted models including SES and other prognostic determinants in the consolidation period (figures 2 and 3 of the supplementary data).
Effect of the implementation of the HF program across SES categoriesMultivariate adjusted Cox proportional hazards models considering the complete study period between January 1, 2015 and December 31, 2019 showed that patients in the HUB-DELTA area in the consolidation period (2018-2019) had a significantly reduced risk of clinically related readmission (HR, 0.757; 95%CI, 0.688-0.833), HF readmission (HR, 0.693; 95%CI, 0.616-0.780), and all-cause death (HR, 0.709; 95%CI, 0.631-0.796) compared with Catalan patients with HF in 2015-2016 and this effect was independent of the level of SES (table 5 of the supplementary data).
Improvements in the risk of clinically-related hospitalizations, HF hospitalizations and all-cause mortality were observed in the HUB-DELTA area compared with the rest of CatSalut during the consolidation period in both SES strata (table 4 and figure 1, and figures 4-8 of the supplementary data). Moreover, in additional adjusted Cox proportional hazards analyses, the interaction term SES strata by health care area was not statistically significant in the consolidations period for all-cause mortality ([health care area*SES strata]:HR, 1.025; 95%CI, 0.731-1.439; P=.884), for clinically-related readmissions ([health care area*SES strata]:HR, 1.049; 95%CI, 0.806-1.366; P=.721), or for HF hospitalizations ([health care area*SES strata]: HR, 1.139, 95%CI, 0.808-1.605; P=.459). Similar results for the interaction terms were observed in the preimplementation and transition periods (table 6 of the supplementary data).
Multivariable (adjusted) Cox proportional models evaluating the impact on clinical outcomes across years and predefined time periods according to health care setting (HUB-DELTA area vs the rest of CatSalut) and stratified by SES
HUB-DELTA Area vs rest of CatSalut | ||||
---|---|---|---|---|
SES | Period | HR | 95%CI | P |
All-cause death | ||||
Medium or high SES | 2015-2016 | 0.929 | 0.789-1.095 | .381 |
2017 | 1.128 | 0.859-1.482 | .386 | |
2018-2019 | 0.832 | 0.607-1.141 | .255 | |
Low or very low SES | 2015-2016 | 0.921 | 0.861-0.985 | .016 |
2017 | 0.865 | 0.770-0.971 | .014 | |
2018-2019 | 0.835 | 0.737-0.947 | .005 | |
Clinically-related readmission | ||||
Medium or high SES | 2015-2016 | 1.292 | 1.119-1.492 | .000 |
2017 | 1.199 | 0.934-1.538 | .154 | |
2018-2019 | 0.880 | 0.690-1.122 | .302 | |
Low or very low SES | 2015-2016 | 1.173 | 1.101-1.249 | .000 |
2017 | 1.134 | 1.025-1.253 | .015 | |
2018-2019 | 0.908 | 0.818-1.008 | .071 | |
HF readmission | ||||
Medium or high SES | 2015-2016 | 1.494 | 1.269-1.759 | .000 |
2017 | 1.105 | 0.802-1.522 | .542 | |
2018-2019 | 0.792 | 0.575-1.090 | .153 | |
Low or very low SES | 2015-2016 | 1.281 | 1.193-1.376 | .000 |
2017 | 1.271 | 1.134-1.425 | .000 | |
2018-2019 | 0.871 | 0.767-0.990 | .034 |
SES, socioeconomic status.
Models were adjusted for SES, sex, age, previous hospitalization, morbidity index (GMA: associated morbidity groups) and time since diagnosis of heart failure.
Interestingly, the size effect was notably more pronounced in the medium or high SES stratum. In this stratum, the effect ranged from HR, 0.559; 95%CI, 0.345-0.904; P=.018 for clinically-related readmission to 0.407, 95%CI, 0.202-0.819; P=.012 for HF readmission in 2019. On the other hand, size effects were substantially lower among the low or very low SES stratum: the best results were obtained in 2019 for clinically-related readmission HR in 2018 (HR, 0.861; 95%CI, 0.760-0.976; P=.019) and in 2018 for HF hospitalization (HR, 0.818; 95%CI, 0.702-0.954; P=.010).
A significant beneficial effect in the HUB-DELTA health care area compared with the rest of CatSalut was observed for all-cause death in all time periods only in the low or very low SES stratum. As shown in table 4 and figure 1; and figures 9 and 10 of the supplementary data, these effects were more pronounced in the consolidation period (HR, 0.835; 95%CI, 0.737-0.947; P=.005). Within the medium or high SES stratum, the risk of death tended to be lower among patients in the HUB-DELTA area than in the rest of CatSalut in a similar magnitude to that observed for patients with low or very low SES. However, this difference was not statistically significant (HR, 0.832; 95%CI, 0.607-1.141; P=.255) in any period or year.
DISCUSSIONIn this study, we have shown that SES is an independent predictor of mortality, clinically-related hospitalization, and HF hospitalization in patients with chronic HF in a health care system with universal coverage. We have also shown that the implementation of an intensive transitional care nurse-based HF management program integrating hospital, primary care, and community resources for patients with HF at a high risk of events translated into benefits in outcomes regardless of the SES of patients exposed to the program. Interestingly, the benefit in terms of hospitalization was observed in all SES strata but the size effect was more prominent among patients with medium or high SES. The benefit of the program in terms of mortality was of similar magnitude across SES strata but was only statistically significant among patients with low or very low SES.
The findings of the present work are unique since this is the first study to evaluate the impact of SES on the effectiveness of integrated HF programs in terms of hard endpoints such as mortality, HF hospitalization and clinically-related hospitalization using population-based approaches and real-world data. Our findings will help to pave the way to define new components of care and interventions to be implemented to meet the needs of patients according to their SES.
Few previous studies have analyzed the influence of SES on the results of managed care of chronic conditions.16 Most of them have focused on assessing the effect of SES on self-management support interventions in relation to participation, retention, and postintervention outcomes. In those studies, low SES was associated with low engagement and low retention of patients in managed care programs for chronic conditions. Studies evaluating postintervention outcomes according to SES level focused on clinical, behavioral, or other specified outcomes following self-management support interventions. Only 1 study evaluated the effectiveness of intensive self-care training on reducing hospitalization for HF and analyzed the benefits by SES strata defined according to literacy level.31 In that study, intensive self-care strategy did not improve overall clinical outcomes. However, patients with low literacy appeared to benefit more from multisession interventions than those with higher literacy. These benefits were observed for hospitalization but not for mortality. In that study, the only component of intervention was a self-care strategy, while other important components of integrated care were not tested. In addition, the study included a limited sample size of 605 ambulatory patients with reduced ejection fraction, and excluded patients with psychosocial limitations. Importantly, SES was classified solely on the basis of the patients’ literacy status of patients and did not include their income. Moreover, due to the design (selection bias associated with any randomized controlled trial) and the health care context of the study (health care system without universal coverage), its results cannot be easy translated into real-world practice.
Many of the limitations and knowledge gaps observed in previous studies have been addressed in our study. First, we conducted a population-based evaluation to avoid biased patient selection and analyzed 77 554 consecutive HF patients. All consecutive patients fulfilling the inclusion criteria in a whole country were included regardless of left-ventricular ejection fraction, psychosocial limitations, age, or other conditions. Second, the natural experiment design allowed us to gauge the true effectiveness of the implemented program and the effect according to SES in a pragmatic way and using real-world data in the context of a public health care system with universal coverage. The implementation was conducted in a well-defined integrated health care area of 209 255 inhabitants during a predetermined period. Third, we focused our evaluation on the transition of care that defines the most vulnerable phase of patients with HF: the postdischarge period. Fourth, we implemented several bundles of interventions in addition to self-care strategies. This allowed us to deliver other components of care that have been proven to be effective in these patients and that better reproduce a real-world implementation of integrated care.10,11,13,14,28,29 Finally, we classified patients into SES strata according to individual income, providing more specific information on economic status than level of literacy in a country where there is also universal coverage for education. Interestingly, in contrast with previous studies,31 the benefits observed with the implementation of a comprehensive HF program extended beyond improvement in clinically-related and HF hospitalization but also encompassed improvement in all-cause mortality.
The aim of the study was to evaluate the impact of SES in the context of a comprehensive, multidisciplinary HF model integrating the HF care of patients between hospital and primary care. The benefits of this model, based on interdisciplinary, multilevel, integrated interventions around transitions of care in patients with HF, have previously been published by our group.6,7 The new program applied additional innovations and components of care on top of those already recommended in clinical guidelines.10,14,32 These included a proactive search and inclusion of patients into the clinical pathway (universal detection), an enhanced discharge coordination, improved early postdischarge contact, extension of structured follow-up pathways of patients in all care settings (hospital-based ambulatory HF clinic, primary care offices, and home based care), shared electronic care plans between hospital and primary care, motivational interview-based nurse interventions and robust key performance indicator monitoring of the implementation. Of note, our study demonstrated that the implementation of our enhanced transitional care model benefits patients regardless of their SES.
Low SES has been largely associated with poor outcomes in patients with HF and in other clinical contexts.3 One of the factors that may explain these findings include the limitations in access to health care services in countries without universal health coverage.33 However, these associations have also been reported in countries with robust public universal health system coverage.3 Additional explanatory factors associated with low SES include the clustering of cardiovascular risk factors and unhealthy habits,34,35 reduced psychosocial support,36 worse recovery after an acute event,37 and housing in service-deprived areas with more air pollution.38
Our study leaves several unanswered questions. First, the interventions and/or components of care needed to close the care gap for more effective prevention of hospitalization between SES strata are unknown. Second, there is also limited information on the factors associated with low or very low SES that may interfere with the engagement, retention, and clinical outcomes of patients in disease management programs for chronic conditions. Thus, future research is required to understand the drivers linked to an attenuated effectiveness of HF programs in these patients.
LimitationsThis study has some limitations. First, the use of administrative health care databases may entail a risk of underrecording medical conditions such as chronic HF. However, nondifferential underrecording of chronic HF across income strata and in different health care areas are unlikely to explain the observed associations between SES and outcomes.
Second, by design, natural experiments obtain data retrospectively. This design has limited ability to establish causality. However, natural experiments allow a more realistic intention-to-treat evaluation of interventions and are free of the main drawbacks of open-label clinical trials.
Third, we used individual annual income as a proxy for SES in our analysis. However, SES is a complicated construct that also incorporates factors like education, employment status, social support, and marital status, most of which were absent from the CHSS database. However, individual annual income is a factor used in our health care system to calculate pharmaceutical copayments and it assists in determining the specific and general health care and social needs to be provided individually from public social services in Catalonia. Further research is required to determine whether wealth itself is the underlying cause of the observed relationships or whether it serves as a marker for other SES characteristics that may have a more immediate impact on outcomes and use of health care resources.3
CONCLUSIONSIn this study, we have shown that SES is an independent predictor of mortality, clinically-related hospitalization, and HF hospitalization in patients with chronic HF in a health system with universal coverage. We have also shown that the implementation of an HF management program improves clinical outcomes across SES strata. However, the size effect in the prevention of hospitalization is more pronounced among patients with medium or high SES.
FUNDINGThis research received no external funding.
AUTHORS’ CONTRIBUTIONSConceptualization, C. Capdevila Aguilera, E. Vela Vallespín, M. Clèries Escayola, C. Enjuanes Grau, and J. Comín-Colet; methodology, C .Capdevila Aguilera, E. Vela Vallespín, M. Clèries Escayola, C. Enjuanes Grau, and J. Comín-Colet; validation, E. Vela Vallespín, M. Clèries Escayola, C. Enjuanes Grau, and J. Comín-Colet; formal analysis, E. Vela Vallespin, M. Clèries Escayola, D. Monterde Prat, J. Piera Jiménez, and J. Comín-Colet; resources, C. Capdevila Aguilera, C. Enjuanes Grau, and J. Comín-Colet; data curation, E. Vela Vallespín, M. Clèries Escayola, D. Monterde; J. Piera., writing—original draft preparation, C. Capdevila Aguilera, E. Vela Vallespín, C. Enjuanes Grau, and J. Comín-Colet; writing—review and editing, C. Capdevila Aguilera, E. Vela Vallespín, M. Clèries Escayola, S. Yun Viladomat, C. Fernández Solana, L. Alcober Morte, D. Monterde Prat, E. Hidalgo Quirós, E. Calero-Molina, N. José Bazán, P. Moliner Borja, J. Piera Jiménez, M. Ruiz Muñoz, X. Corbella Virós, S. Jiménez-Marrero, A. Garay Melero, R. Ramos Polo, L. Alcoberro Torres, A. Pons Riverola, C. Enjuanes Grau, and J. Comín-Colet; supervision, J. Comín-Colet; project administration, C. Capdevila Aguilera, and J. Comín-Colet; funding acquisition (APC), J. Comín-Colet. All authors agree to be accountable for all aspects of the work in ensuring that questions related to the accuracy or integrity of any part of the work have been appropriately investigated and resolved.
CONFLICTS OF INTERESTThe authors declare that they have no conflicts of interest relevant to the content of this manuscript.
- -
Low socioeconomic status is associated with poor clinical outcomes in patients with heart failure.
- -
Multidisciplinary heart failure care in structured heart failure programs has been demonstrated to improve clinical outcomes in patients at high risk of readmission.
- -
It is currently unclear whether intensive managed care of patients with heart failure in in the setting of heart failure programs can provide benefits regardless of their socioeconomic status.
- -
Confirms that low socioeconomic status is associated with poor outcomes in patients with heart failure in health care systems with universal coverage.
- -
Demonstrates that the implementation of multidisciplinary heart failure programs integrating community, primary care, and hospital resources is feasible, that these programs can reduce mortality and morbidity in high-risk patients with heart failure at the population level.
- -
Shows that the benefits of multidisciplinary, transitional, and integrated care in high-risk patients with heart failure are independent of the patients’ socioeconomic status.
The authors would like to acknowledge the contribution of all members of the working group of the South Metropolitan Barcelona Community Heart Failure Program. We also thank CERCA Program/Generalitat de Catalunya for institutional support.
Supplementary data associated with this article can be found in the online version, at https://doi.org/10.1016/j.rec.2023.03.009